Sponsored by:
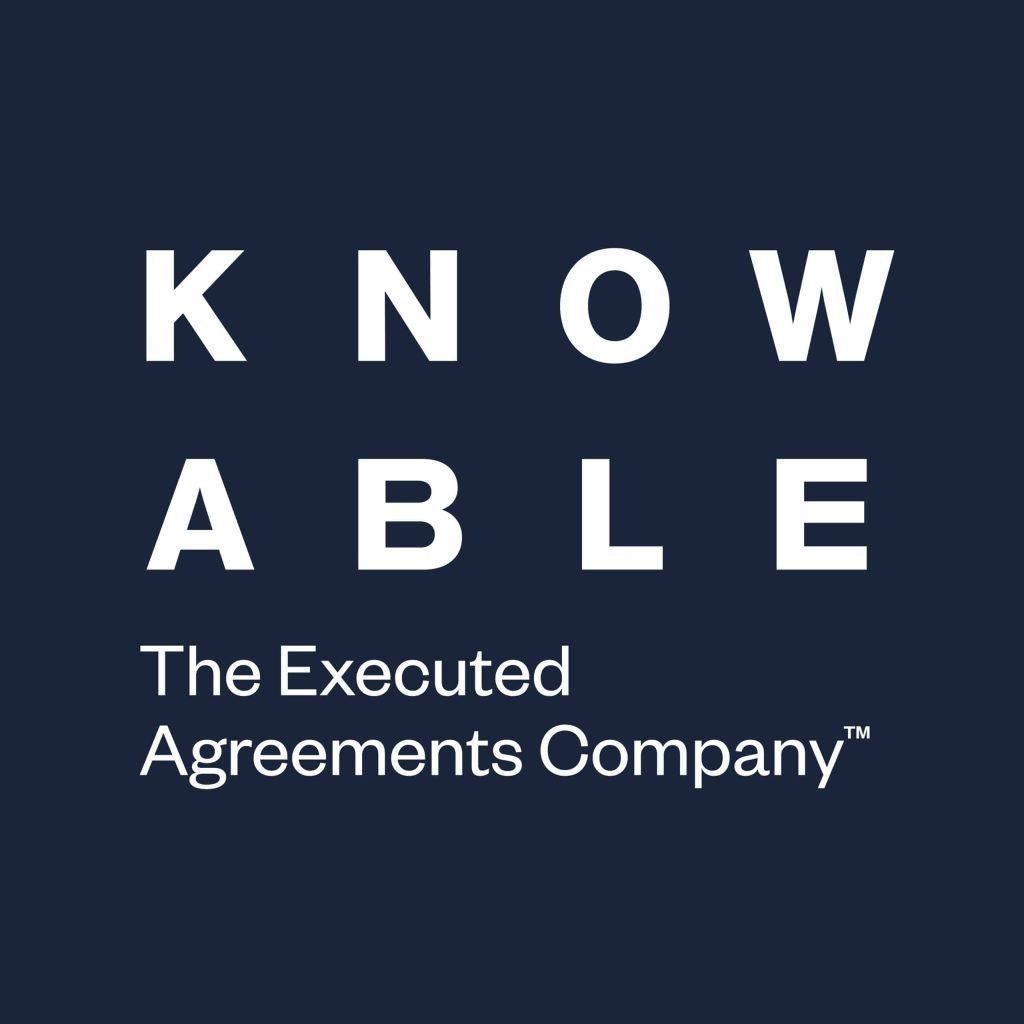
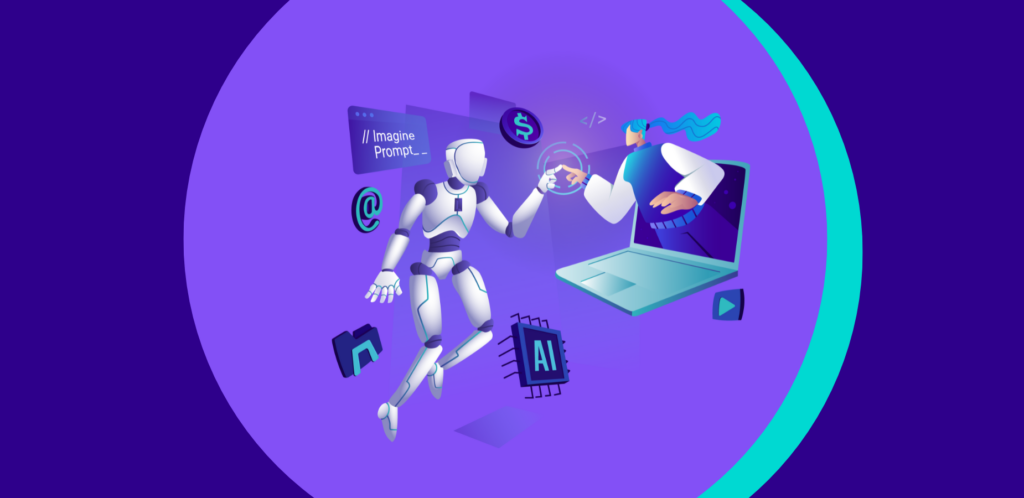
- December 11, 2023
- Share:
7 Questions to Ask Your Legal AI Tech Provider
With the proliferation of AI-based tools for legal at a seemingly all-time high, a pressing concern for most legal teams is — how can I validate the effectiveness of Legal AI tools while safeguarding the confidentiality and security of my company and its data? Or, put more simply, how do I pick the right solution(s) for my team and organization?
Having worked in legal tech for 13 years, I’ve had numerous conversations with lawyers and legal operations professionals about the various challenges they hope technology can solve, many of which haven’t changed for the last 10 to 15 years (such as locating contracts). But despite its great potential, using AI-based legal solutions requires adjusting our expectations or at least better understanding new trade-offs.
At Ravel, the company I co-founded out of Stanford Law School in 2012 before joining Knowable, we always thought the best AI was AI you didn’t know was there. We set out with the goal of helping lawyers solve the kind of problems that only software, cloud computing, and, yes, AI could help solve. We always believed the attorneys didn’t need to know how the software was made, just that they could rely on it, but therein lies the crux of the issue. How can you discern which tools are right for your organization and best suited for a specific task? And how do you manage potential new risks, whether it be intellectual property, copyright, or quality?
Historically, the answer to those two questions was pretty simple. We would test the product and evaluate the result. If the answers were good enough, we’d move forward with a plan to iterate and improve over time. But that was before 2023 (well, really 2022) and the mass market advent of Large Language Models (LLMs) like GPT, Bard, and Anthropic.
Today, we must ask different questions to get to the right answer. During the inaugural Harvard Law AI Summit, a leading specialist in artificial intelligence (under the condition of anonymity as per the Summit’s rules of attendance) pointed out that while we don’t understand how LLMs work, humans have often used technology we don’t understand, such as cooking. We cooked food and made all kinds of dishes for over 10,000 years before understanding the underlying science. Trial and error were all we had. But as legal experts, is trial and error acceptable? Can we really afford that level of risk?
7 Critical Questions
In reality, we don’t have a choice. AI is not coming; it’s already here. Here are seven questions to ask your potential Legal AI provider to help you determine if a particular AI solution is suitable for your organizational needs and, more importantly, to identify which provider you are willing to entrust with a certain level of risk.
1. Is your IP protected?
This question seems fundamental, but the consequences are real. Remember the news story about Samsung engineers who fed proprietary source code into ChatGPT that OpenAI then used as training data to answer other users’ questions? Each time you ask a model to do something for you, it’s curating information and delivering an output from numerous sources. Despite having millions or trillions or more data points, open and public models are learning from every input and, in some cases, can serve up proprietary information given the right prompts.
There are ongoing debates about the legal risk associated with AI and its potential infringement, but the bottom line is your IP needs to be protected. Ask your provider if you have the right to use the AI’s output or if it belongs to someone else from whom you must seek permission. Also, ask whether the provider owns the model and has copyright in the answers. Most companies today state that you have the right to use the answers, but you should confirm before selecting a provider. Lastly, ask if you are indemnified in the event of copyright infringement. In a world of trial and error, you have a right to know.
2. What models are being used? And are they open or restricted?
This is a variation of question one, but it’s an essential component of your evaluation. The point highlights three types of LLM models that you should understand. Models like GPT are open, public, and continuously learning from everything it ingests, updating its knowledge and making it available to anyone using the tool. Forked models are customized based on an open-source model from a fixed point in time, only answering questions based on its existing knowledge set. Proprietary models are developed from scratch in-house and not shared externally (or can be built off a forked model). This is the ultimate build vs. buy question, with the latter requiring a heavier lift to keep the training data up to date. (see question #5)
You should also ask if the models being used are open or restricted. Often referred to as “walling,” models can be deployed via commercial agreements with AWS or MS Azure (and hence are “walled”), but they can also be deployed without commercial protections. Pending your risk tolerance, you may be more or less comfortable with a walled or open solution.
Importantly, providers should be comfortable sharing some details about their infrastructure. While they may not reveal the specifics (as that is undoubtedly part of the company’s IP), it is not controversial to tell you if they are multi-model or single-model, nor is sharing specific names, like OpenAI, Anthropic, Bard, or BERT. Armed with this knowledge, you can engage in proper research and better understand the pros and cons of each LLM when comparing vendors and deciding whether to build one yourself.
3. What if PII is placed into the model?
What happens if you input someone’s address or upload a document that contains personally identifiable information (PII)? Revisit questions one and two, as this could create issues with GDPR or CCPA for you and the provider, increasing your company’s risk and liability. You may need to revisit or create policies and ensure enforcement, much as you do today around the use of PII.
4. How do you prevent “model drift?”
Over time, the results of AI models “drift,” which means the results or the outputs from a model or solution change in one of two ways.
-
- Data Drift: When the input data changes significantly, it is considered “data drift.” For example, suppose the model is trained on older contracts, and you implement a new contracting template. Consequently, the model may be unable to predict results as well on the new documents.
- Concept Drift: There is also a possibility of “concept drift.” For example, if the meaning of a term changes (like a contracting term), the dataset becomes more expansive and, thus, less effective as we use it over time.
In both cases, the model struggles to adapt to new, unexpected changes, and hence the results change. Additionally, the company you are working with may switch to an entirely different model or a different version of a model. For better or worse, the results are likely going to be different. How will you know when that happens and are the differences acceptable?
5. Do you do the training and evaluation yourself?
Especially in a world of LLMs, training is increasingly being handed over to the model creators. In these situations, the model is no longer getting better based on specific “gold standard” data but on what it has been trained on writ large. Depending on your use case, this may be sufficient, but you may want an experienced provider to train your model to increase precision and accuracy.
With training comes evaluation — a costly and challenging endeavor. It is imperative to know how models are performing if you are going to use their outputs. Ask your provider who will be responsible for the evaluation. If the answer is you, analyze the time and resources needed to put teams in place that specialize in model evaluation and ask yourself whether you are ready to assume that cost.
6. What data do you get back?
If you use a model to extract data such as redlines, drafts, or answers to contract questions, understand what the AI tool will ultimately deliver. Is it just a blurb of text or a full string and conversation? Ask your vendor to provide real-world examples of what you will receive and in what form based on your contract data set as part of your proof of value (POV).
7. Do you guarantee accuracy?
Lastly, and likely often most importantly for attorneys and their legal teams, is how trustworthy is the output? Accuracy is a critical success metric. Many companies hedge by saying, “The model learns the more you use it,” or “As you correct the output, the model gets better.” This is highly unlikely for the reasons mentioned in questions four and five since your input data will change over time, which may improve the model and sometimes confuse it. Furthermore, there are diminishing returns to training – at a certain point, the benefits become incremental and likely not noticeable. In the end, the outcomes of AI-only tools will be unpredictable, which will erode trust. This is why it is still critical to have humans-in-the-loop (HITL) to reduce risk and ensure accuracy.
What’s Next?
Amidst all this uncertainty, what can you do? The good news is traditional approaches to minimizing risk hold up.
-
- Step 1: Ask all the questions above. Don’t avoid them.
- Step 2: Ensure your IP is protected contractually.
- Step 3: Ask the company to be open about the models they use and their overall approach to training and evaluation.
- Step 4: Ask about accuracy and how it will be continuously monitored and validated. (Hint: HITL should be a core part of this process.)
I am quite frankly very bullish about AI for legal. Thanks to technology, I have seen attorneys accomplish previously impossible tasks and have great outcomes. I’ve also seen lots of companies overpromise and under-deliver. My former Ravel co-founder Daniel Lewis recently made the point that lawyers don’t all need to become prompt engineers to get the benefits of AI, but we do need to ask questions and be smart about who we work with.
Have questions about how Knowable thinks about ML/AI in post-signature contract management? Visit knowable.com.
By Nik Reed, SVP, Product and Research & Development, Knowable
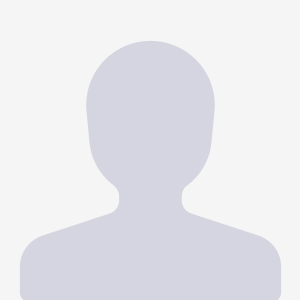